Why Should You Care About Algorithmic Bias in 2025?
Q: Can a machine be racist or sexist?
A: In a phrase, sure—although not in the best way people are. Machines ruled by algorithms can perpetuate and even amplify human biases if the info they’re fed is skewed.
As we rapidly approach 2025, this issue becomes increasingly important as AI systems are making more decisions that impact our lives, from job screenings to mortgage approvals.
The hazard lies in the subtlety with which these biases may be woven into the material of our everyday interactions, typically going unnoticed till they trigger significant harm to susceptible populations.
Yes. In 2025, algorithms form hiring, healthcare, finance, and justice programs; however, hidden biases in their design threaten equity, fairness, and democracy.
To combat these challenges, the call for transparency and accountability in AI programs has never been stronger. Ethical frameworks and regulatory guidelines are being established to ensure that AI personalization benefits the greater good instead of reinforcing existing inequalities.
It is crucial that these programs are audited for bias and that the datasets they study from are representative of the various populations they influence. Only via vigilant oversight and inclusive design can we harness the ability of AI to reinforce, rather than undermine, our collective well-being.
Imagine losing a mortgage, a job, or parole because an algorithm got it wrong. This isn’t science fiction—it’s happening now. By 2025, unchecked algorithmic bias could worsen societal inequalities.
From facial recognition misidentifying folks of colour to AI hiring instruments favoring male candidates, bias lurks in code. This article exposes the myths, dangers, and options you want to know.
Understanding Algorithmic Bias: Key Definitions
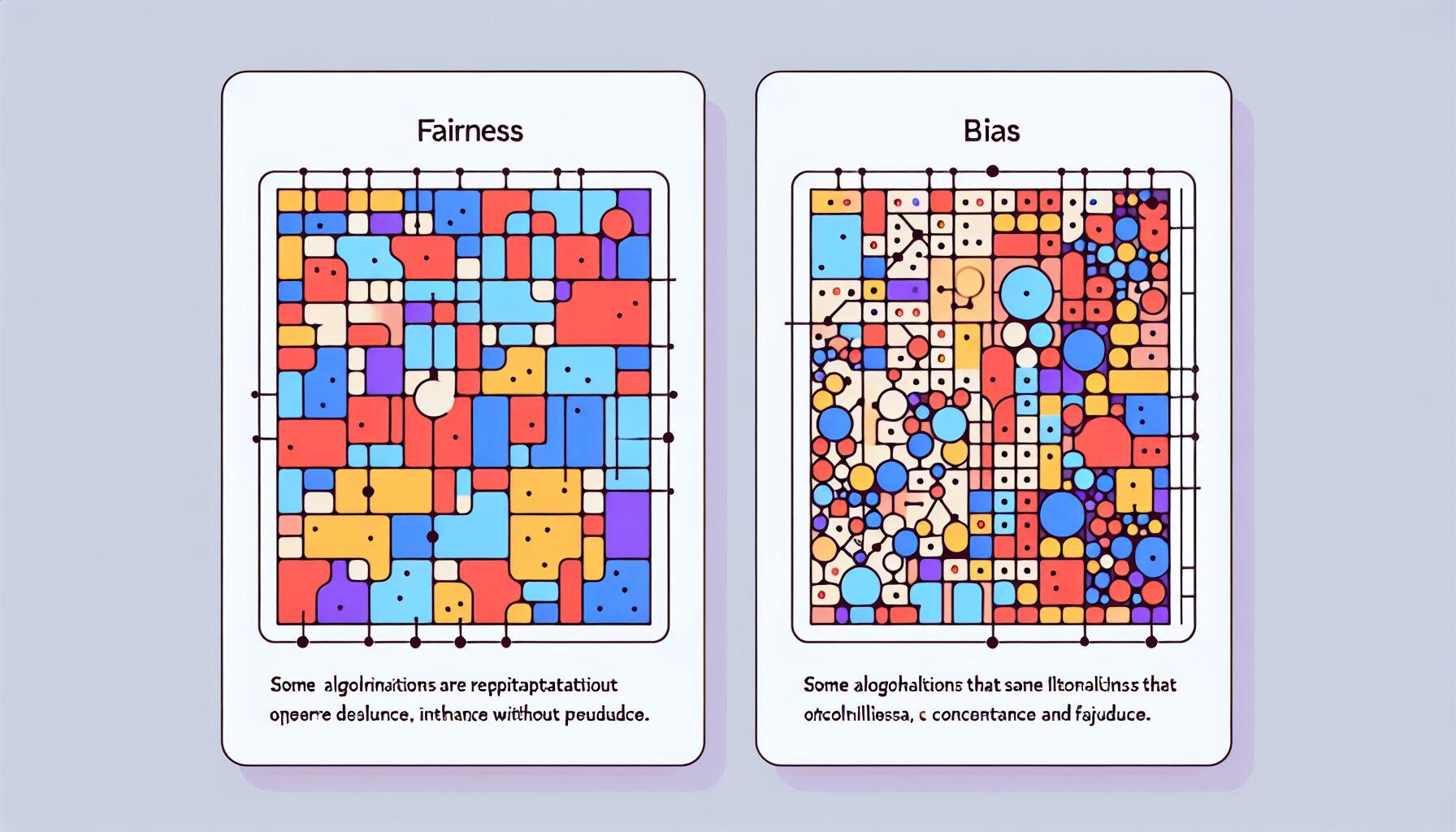
Algorithmic bias arises when a computer system mirrors the unconscious values, prejudices, or assumptions embedded by its creators, the data it analyzes, or the societal frameworks it operates within. Such biases often manifest in ways that disproportionately impact marginalized communities, resulting in adverse and inequitable outcomes.
By examining the mechanics of algorithmic decision-making, we can identify where biases may originate and how they spread through systems that appear neutral.
Addressing these issues requires a multifaceted approach, including the involvement of diverse groups in AI development, thorough bias audits, and continuous public discussions about the ethical use of technology.
Algorithmic bias refers to systematic errors in AI programs that create unfair outcomes for particular teams. Rooted in biased coaching information, flawed design, or human oversight, these biases replicate real-world discrimination at scale.
Why It Matters in 2025:
1: As we delve further into the era of AI personalization, the urgency of addressing algorithmic bias has never been greater. By 2025, the widespread integration of AI into everyday life means these biases could significantly influence individual opportunities and societal frameworks.
From job recruitment to credit scoring and even the content we consume daily, AI’s impact is ubiquitous, turning the quest for ethical algorithms into both a technical challenge and a moral necessity. By 2025, 85% of enterprises are expected to deploy AI (Gartner).
2: As we embrace the dawn of this AI-driven era, personalization has become a vital element of its transformative power. By customizing experiences to individual preferences, behaviors, and needs, AI personalization is revolutionizing the way we interact with technology, products, and services.
However, this hyper-customization raises vital questions on privacy, information safety, and the potential for algorithmic bias that would perpetuate inequalities.
As we use AI to create more personalized experiences, it’s crucial to set strong systems that safeguard individual rights and promote fairness for everyone. Worldwide spending on AI regulation is expected to hit $6.4 billion (IDC).
3: As AI personalization becomes more common, it’s important to ensure transparency in how algorithms make decisions affecting people. Businesses must be accountable for the data they collect and how they use it to customize experiences, providing clear communication to users about the insights shaping their interactions.
AI programs should use diverse datasets to reduce biases that could lead to discrimination, ensuring personalization improves user experiences while maintaining fairness and inclusivity. In healthcare, bias in AI may lead to the misdiagnosis of 12 million patients each year (WHO).
The Evolution of Algorithmic Bias: From 2023 to 2025
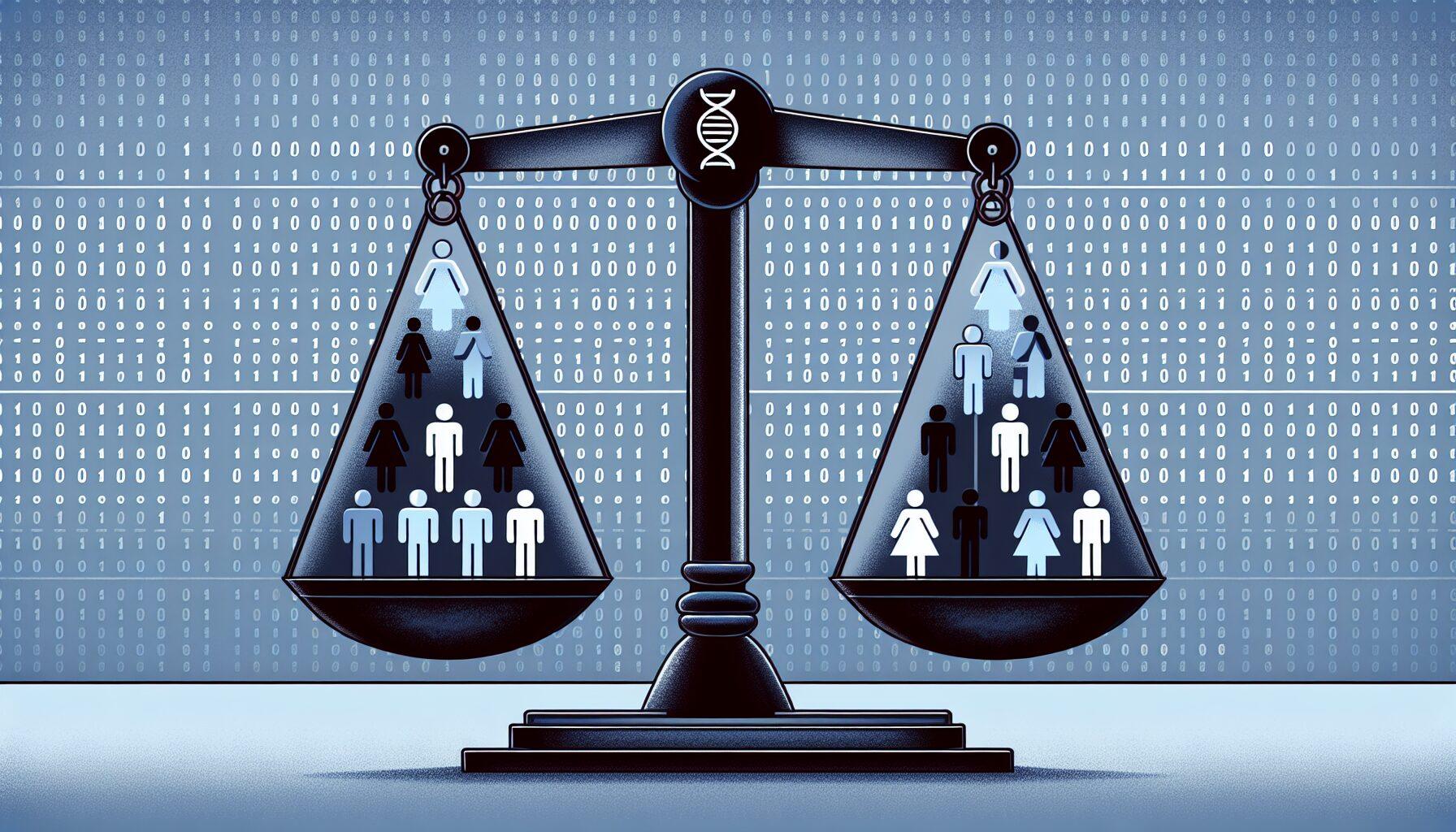
1. How Do Biases Creep Into AI Systems? Data Bias: Data bias occurs when the datasets used to train AI systems fail to accurately represent the diverse populations they aim to serve. This may result from historical data being influenced by societal inequalities or from an inadequate sampling of data points.
When a healthcare AI is primarily trained on data from a single demographic group, it may face challenges in accurately diagnosing conditions that manifest differently or are more prevalent in other groups. This can result in unequal treatment outcomes. The lack of diverse representation in training data further exacerbates these disparities.
2: Design Bias: Design bias in AI personalization emerges when algorithms are crafted with inherent assumptions or preferences that might not be universally relevant. This may result in programs that favor certain personal behaviors or traits, inadvertently marginalizing those that don’t match the prescribed model.
To address this, it is crucial to incorporate diverse perspectives throughout the enhancement process and establish robust bias detection and correction mechanisms. This ensures that personalization technologies effectively serve and empower individuals. Misguided objectives, such as prioritizing profit over equity, must be avoided.
3: Deployment Bias: To address deployment bias, continuously test AI systems in real-world situations. This involves tracking performance metrics but also gathering feedback from users across various demographics.
By taking these steps, developers can identify and address any unforeseen consequences or inconsistencies in service quality, ensuring the principles of fairness and inclusivity in AI personalization are maintained. This also helps prevent misuse in contexts beyond the original intent, such as predictive policing.
Case Study: Predictive policing highlights the challenges of AI personalization. This technology uses data analytics to forecast crime locations or identify individuals more likely to commit crimes. While aimed at improving law enforcement efficiency, it can unintentionally amplify biases in the historical data it uses.
For instance, if a mannequin is skilled on information from neighborhoods with traditionally larger police presence, it might disproportionately goal these areas, irrespective of the particular crime rate.
This creates a risky cycle where some communities face excessive policing driven by predictions instead of solid evidence. This underscores the need for careful oversight and ethical considerations in using AI personalization tools. For example, Amazon’s discarded hiring tool reportedly downgraded resumes mentioning terms like “women’s chess club” (Reuters).
2. What Industries Are Most Vulnerable in 2025?
1: Healthcare: The healthcare business stands at a very precarious intersection of AI personalization, given the delicate nature of medical information and the potential for life-altering selections.
By 2025, the growth of AI in healthcare could lead to highly personalized treatment plans, but it also brings concerns about privacy and the risk of biases in algorithms affecting diagnoses and care decisions.
It is paramount that rules and moral frameworks maintain pace with technological developments to make sure that AI aids rather than hinders equitable entry to healthcare companies. Diagnostic instruments skilled on predominantly white male information.
2: Finance: In the realm of finance, AI personalization is revolutionizing the way people and companies handle their monetary well-being. By leveraging huge quantities of information, AI algorithms can provide tailor-made recommendations on funding methods, risk evaluation, and spending habits, making monetary planning more accessible and personalised than ever earlier than.
This shift not solely empowers customers with personalized monetary insights but additionally challenges conventional banking establishments to adapt and innovate, making certain they continue to be aggressive in an more and more tech-driven market.
However, it’s vital to handle issues relating to information privacy and the potential for algorithmic bias, which might perpetuate current inequalities if left unchecked. Loan algorithms discriminate against low-income ZIP codes.
3: Criminal Justice: In the realm of prison justice, AI personalization has the potential to revolutionize the system by offering extra accurate danger assessments and helping to scale back human bias in sentencing and parole decisions.
However, this similar expertise may also elevate moral questions and issues about equity if not applied with caution. It is crucial to make sure that AI algorithms are clear and recurrently audited for biases that would affect people based mostly on race, gender, or socioeconomic standing, thereby undermining the very justice they seek to reinforce. Predictive policing amplifies racial profiling.
Debunking 3 Myths About Algorithmic Bias
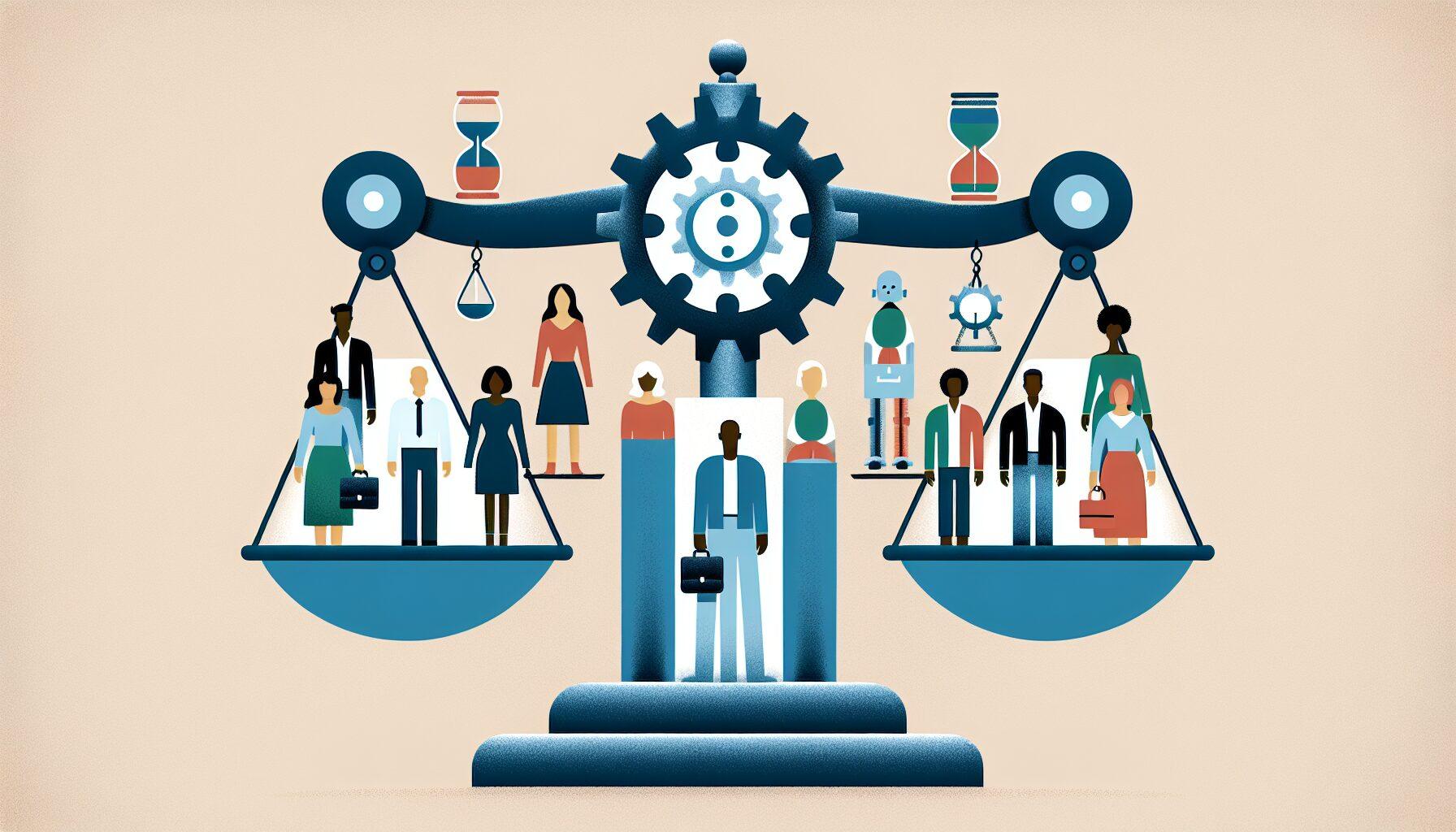
Myth 1: “AI is Neutral Because Math Can’t Be Biased.” Truth: Algorithms replicate their creators’ biases. A 2023 Stanford research discovered that 68% of AI builders admit to prioritizing velocity over ethics.
Myth 2: “More Data Solves Everything.”
Truth: Garbage in, rubbish out. Overrepresented teams skew outcomes (e.g., facial recognition failing darker pores and skin tones).
Myth 3: “Regulation Stifles Innovation.”
Truth: The EU AI Act (2024) mandates transparency, boosting public belief and long-term adoption.
The 2025 Landscape: Emerging Threats & Solutions
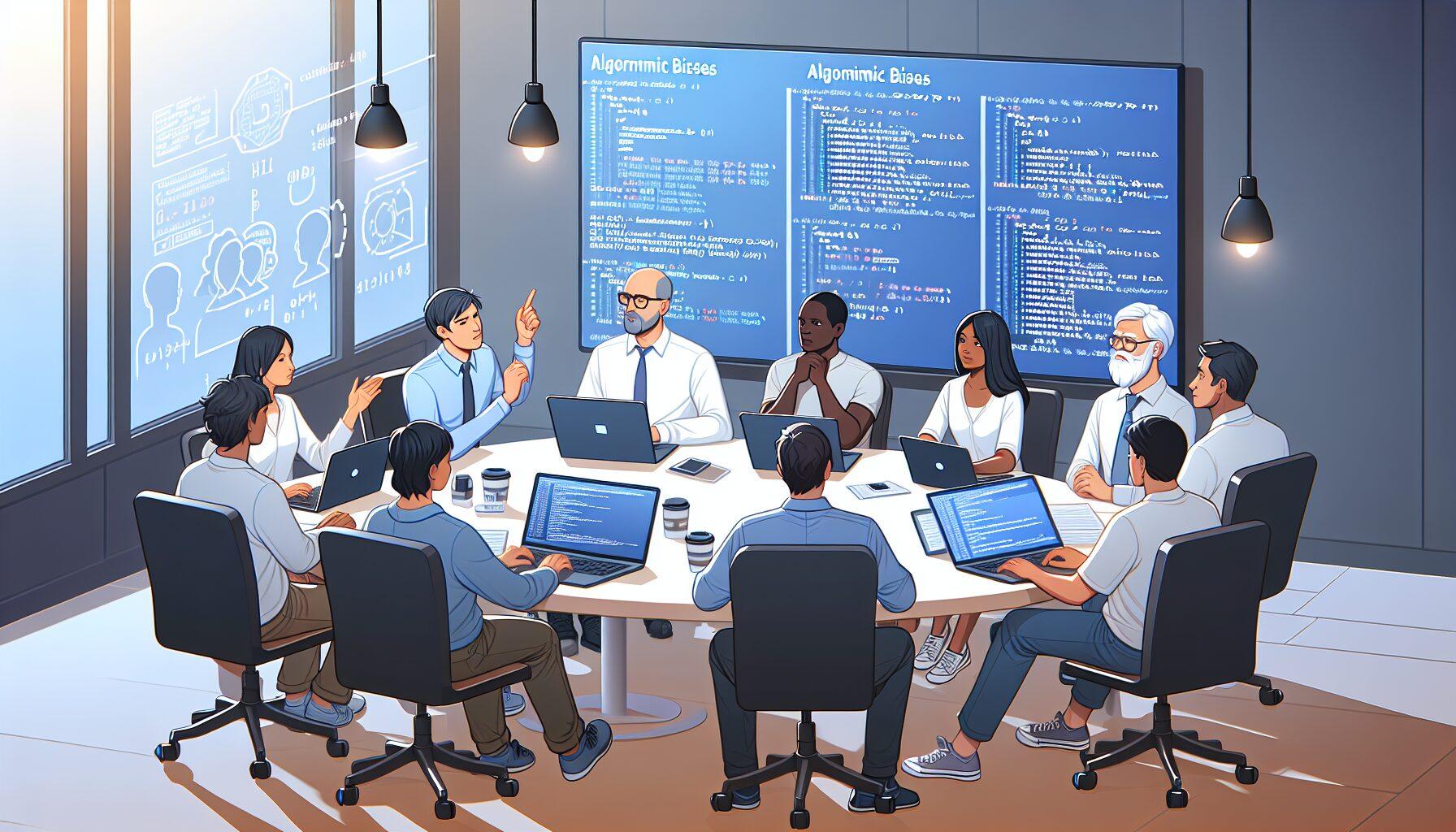
1. Deepfake-Driven Discrimination
As we navigate the ever-evolving digital terrain of 2025, the proliferation of deepfake expertise poses a major risk to private and company integrity.
This subtle type of AI-generated media manipulation will not be solely able to distort the reality in the general public area but additionally has the potential to exacerbate current biases inside AI personalization algorithms.
To mitigate these dangers, builders must incorporate strong moral frameworks and bias-detection mechanisms into their AI programs, making certain that personalization enhances personal experiences with out compromising equity or accuracy. AI-generated content material might weaponize bias, like artificial voices mimicking accents to disclaim customer support.
2. Autonomous Systems & Accountability Gaps
As AI personalization continues to evolve, it is essential to handle the potential accountability gaps that will come up with autonomous programs. When selections are made without human intervention, it becomes difficult to pinpoint the cause for errors or biases.
This requires a sturdy framework that not only oversees AI operations but additionally ensures that there are clear pointers for legal responsibility and redress when these programs fail quickly.
Establishing such protocols might be important in sustaining belief in AI personalization, as customers should really feel assured that they will have recourse in the event of a mishap or injustice perpetuated by an autonomous system. Self-driving automobiles make split-second moral selections (MIT’s Moral Machine experiment highlights cultural biases).
3. Bias in Generative AI
The problem of bias in generative AI isn’t just a technical hurdle but additionally a profound moral concern. Systems skilled on historic information can inadvertently perpetuate stereotypes and discriminatory practices if not rigorously monitored and adjusted.
As builders and stakeholders, we should prioritize the creation of various datasets and implement strong equity metrics to make sure that AI-generated content material displays a balanced and inclusive perspective, mitigating the danger of reinforcing dangerous biases in society. ChatGPT’s 2023 tendency to supply sexist profession recommendation underscores the dangers of scaling unchecked fashions.
Solution Spotlight:
1: Explainable AI (XAI): Explainable AI (XAI) emerges as a vital answer to the problem of opaque decision-making processes inside AI programs. By prioritizing transparency, XAI permits customers to know, believe, and successfully handle AI outputs.
This method not solely helps in figuring out and addressing biases in AI-generated content but additionally empowers stakeholders to make knowledgeable selections based on clear insights into how AI models arrive at their conclusions.
As AI personalization becomes more and more prevalent, the necessity for XAI becomes crucial to ensure moral and equitable AI practices. Tools like IBM’s AI Fairness 360 audit fashion for disparities.
2: Diverse Teams: Building on the inspiration of XAI, fostering various groups in the AI discipline is vital for creating personalization algorithms that can be unbiased and representative of the worldwide inhabitants.
Diversity in AI improvement groups not solely brings quite a lot of views to the table but additionally helps in figuring out and mitigating potential biases that could possibly be embedded in AI programs.
By incorporating people from totally different backgrounds, cultures, and disciplines, AI personalization efforts can profit from a broader understanding of people’s wants, making certain that personalised experiences are inclusive and respectful of the rich tapestry of human variety. Companies with gender-diverse groups cut back bias incidents by 33% (McKinsey).
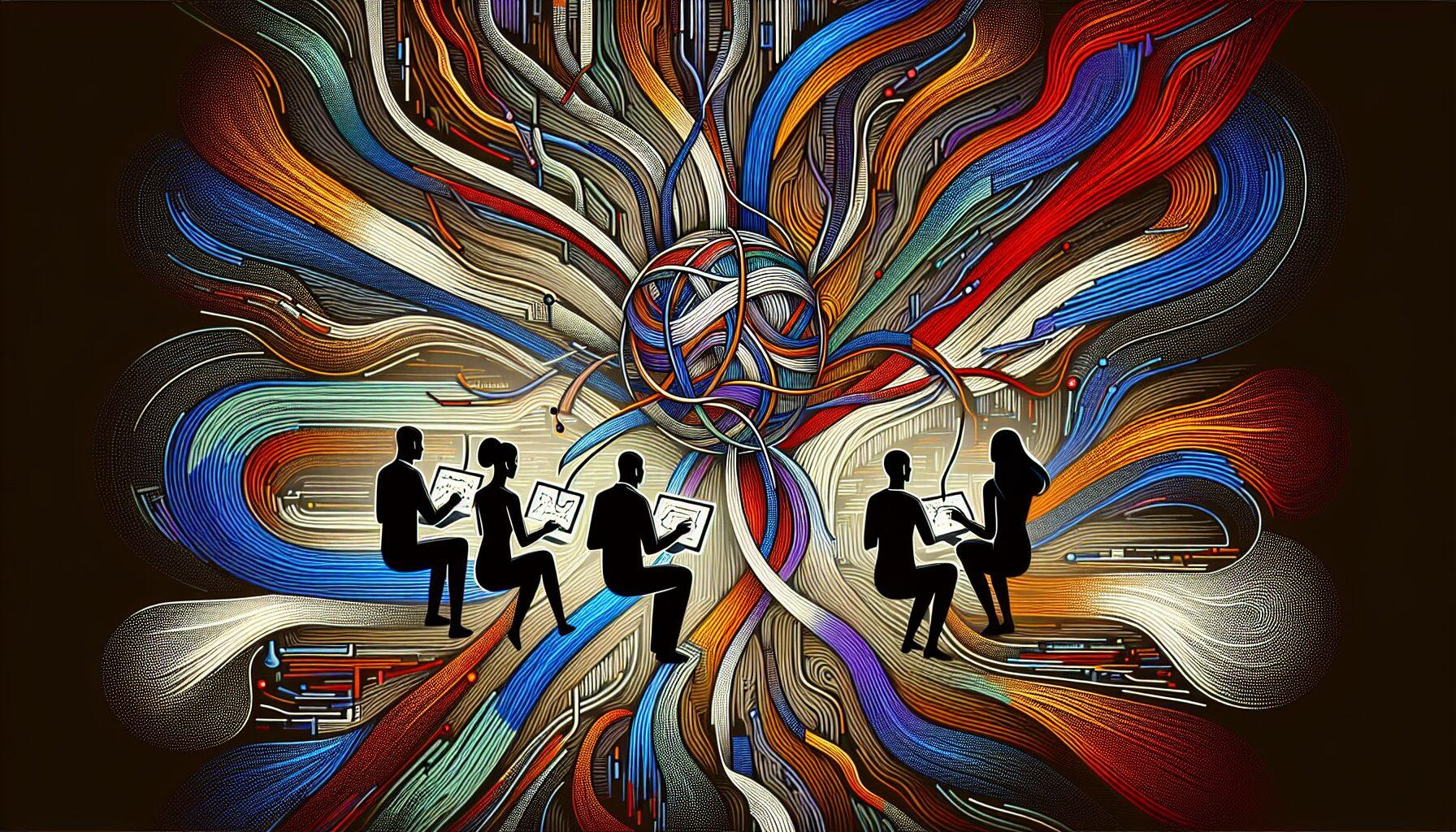
Top 3 Google Searches on Algorithmic Bias (With Quick Answers)
Q1: “How to detect algorithmic bias?” A: Use equity metrics (demographic parity, equalized odds) and audit instruments like Google’s What-If Tool.
Q2: “What legal guidelines regulate AI bias?”
A: EU AI Act (2024), NYC’s AI Hiring Law (2023), and California’s Algorithmic Accountability Act (2023).
Q3: “Can biased AI be fixed?”
A: Yes—by way of re-training information, adversarial debiasing, and human-in-the-loop oversight.
How to Combat Algorithmic Bias: 5 Actionable Strategies
1: Audit Your Data: Ensure that the datasets you employ for coaching your AI programs are as diverse and inclusive as possible. This means not solely gathering information from a variety of sources but additionally critically analyzing it for potential biases that would skew your AI’s decision-making.
Regular audits by impartial third parties may help establish and mitigate these biases earlier than they turn out to be entrenched in the system’s algorithms. Scrutinize datasets for illustration gaps (use IBM’s Fairness Kit).
2: Adopt Ethical Frameworks: 3: Foster Transparency and Accountability: It’s essential to make sure that AI programs aren’t simply black packing containers with inscrutable processes. Implementing explainable AI (XAI) practices could make the decision-making pathways of your AI extra comprehensible to customers and stakeholders.
This transparency fosters belief and permits accountability, because it makes into doable to trace back and perceive the reasoning behind particular AI actions or suggestions.
Furthermore, clear documentation of AI programs and their underlying logic aids in sustaining this transparency over time and through personnel transitions. Follow UNESCO’s AI Ethics Guidelines or Microsoft’s Responsible AI Standard.
3: Demand Transparency: Foster Accountability: As AI personalization turns into extra ingrained in our digital experiences, it is essential to ascertain accountability for the decisions made by these programs. This means not solely making certain that AI operates inside authorized and moral boundaries but also that there’s a clear line of duty when issues go awry.
Companies ought to implement oversight mechanisms, similar to audit trails and influence assessments, to monitor AI conduct and deal with any detrimental outcomes swiftly. By doing so, they construct belief with customers and show a dedication to accountable AI utilization. Pressure distributors to reveal mannequin metrics and determination logic.
4: Diversify Teams: Diversifying groups isn’t just a matter of social duty; it is a strategically crucial step for AI personalization. A staff composed of people with various backgrounds brings a mess of views to the desk, which is essential for creating AI programs that can be inclusive and unbiased.
By making certain variety in gender, race, tradition, and professional expertise, organizations can develop AI options that resonate with a wider audience and keep away from the pitfalls of a homogenous design philosophy that will inadvertently perpetuate systemic biases or overlook important segments of the population. Hire ethicists, sociologists, and marginalized voices in AI improvement.
5: Advocate for Regulation: Embrace Transparency: As AI personalization becomes more and more subtle, builders and corporations need to keep up transparency about how private information is used to tailor experiences.
Users ought to have clear insights into the algorithms that form their digital surroundings, and they need to be capable of managing the extent of personalization they obtain.
By fostering an open dialogue about AI methodologies and their implications, firms can construct belief and make sure that personalization enhances person autonomy moderately than undermining it. Support insurance policies requiring algorithmic influence assessments.
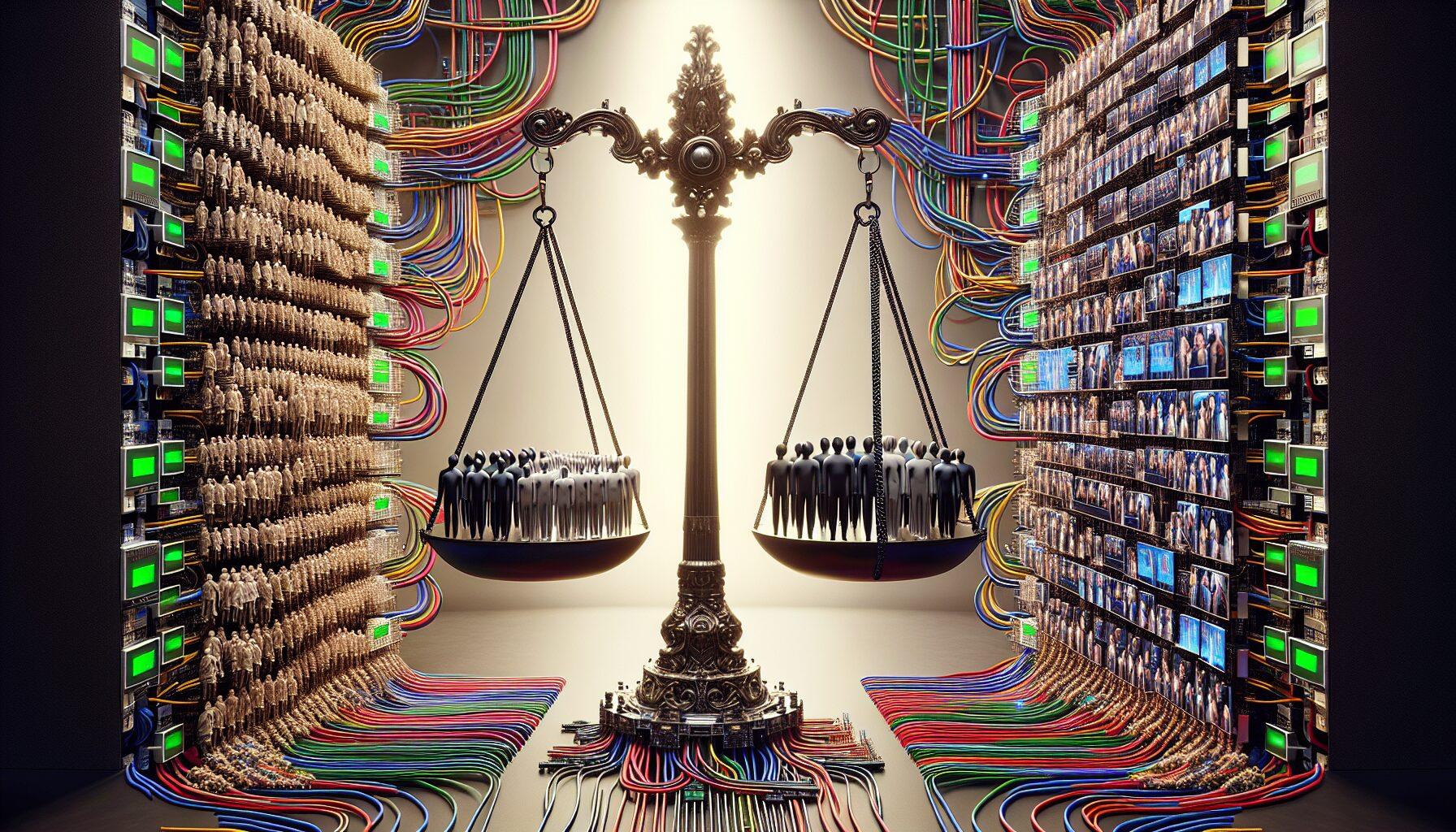
Pro Tip: In this digital age, AI personalization stands as a beacon of tailor-made experiences, promising to ship content material that resonates with the personal preferences and behaviors of customers. However, we must navigate this terrain with an eager sense of moral duty.
To obtain this, companies should prioritize transparency in their AI programs, offering customers with clear insights into how their information is being used and for what functions.
This not solely empowers customers but additionally fosters a way of management over their digital interactions, paving the way for a extra harmonious relationship between AI and its human counterparts.
By doing so, we make sure that AI personalization stays a device for comfort and relevance, not a mechanism for manipulation or privacy erosion. “If you’re a startup, begin small—audit one vital mannequin quarterly.”
3 Essential Tools to Mitigate Bias in 2025
AI Fairness 360 (IBM): Open-source library with 70+ equity metrics. 2. What-If Tool (Google): Visualize mannequin efficiency throughout subgroups. 3. Fairlearn (Microsoft): Assess and enhance model equity in Python.
The Role of Policy: Global Regulations in 2025
Region | Law | Key Requirement |
---|---|---|
EU | AI Act (2024) | Ban high-risk AI in policing/surveillance |
USA | Algorithmic Accountability Act | Mandate bias audits for federal contractors |
China | AI Ethics Guidelines | Require “social duty” in AI improvement |
Expert Quote: “Regulation will not be the enemy—it’s the blueprint for moral innovation.”
—Timnit Gebru, Founder of DAIR Institute
Case Study: How Finland Reduced Hiring Bias by 40%
Building upon this basis of moral AI, personalization applied sciences are taking middle stage, demonstrating the ability of AI to reinforce particular person experiences while adhering to moral requirements.
In Finland, the implementation of AI-driven instruments in the hiring process has not solely streamlined candidate analysis but has additionally considerably curbed unconscious bias, making a extra equitable job market.
By setting a precedent with clear moral pointers, AI personalization may be directed in the direction of fostering inclusivity and equity, thus showcasing the potential of expertise to be a pressure for social good when coupled with accountable governance. In 2024, Finland’s authorities partnered with startups to develop anonymized hiring algorithms. Results:
1: The outcomes from Finland’s modern method of anonymized hiring algorithms have been fairly promising. By stripping away identifiable data that would result in unconscious bias, the AI-driven course has considerably leveled the taking part in discipline for candidates from various backgrounds.
This has not solely elevated the variety of hires throughout numerous industries but has additionally bolstered public belief in artificial intelligence as a device for enhancing fairness in employment practices.
The success of this initiative is a testimony to the ability of AI personalization when it’s rigorously calibrated to prioritize moral outcomes over mere effectiveness. 30% improvement in feminine hires in tech.
2: Building on this momentum, AI personalization will not be solely reshaping the hiring panorama but additionally making certain that variety and inclusion are more than simply buzzwords. By analyzing huge datasets without the inherent biases that may cloud human judgment, AI programs are figuring out and selling certified candidates from a broader spectrum of backgrounds.
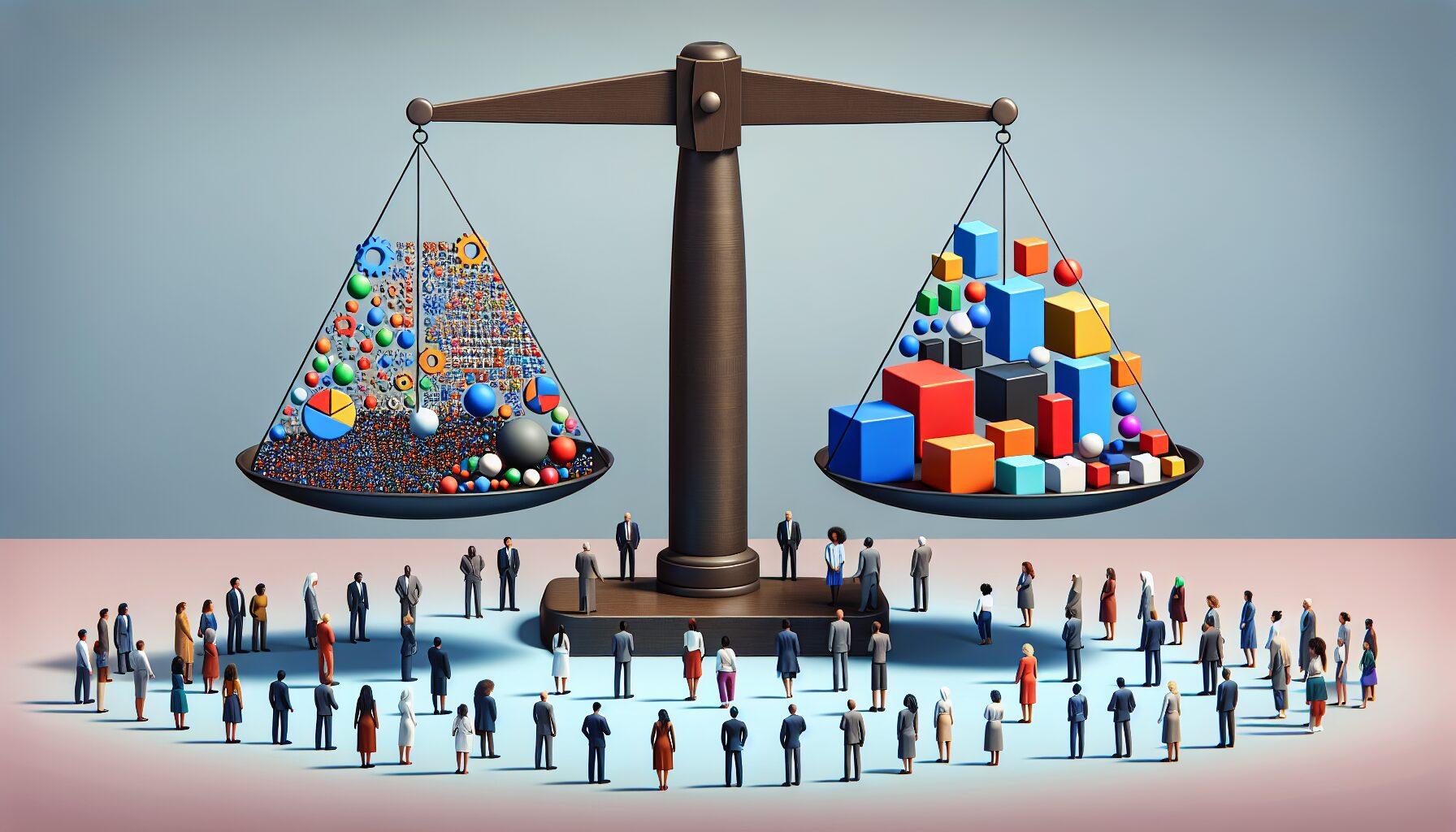
This method is essential in sectors like expertise, the place various views fuel innovation and drive development. As a consequence, the 30% improvement in female hires is only the start of a transformative journey in the direction of a extra balanced and consultant workforce. 22% extra candidates from non-elite universities.
Lesson: Embracing this shift in the direction of inclusivity not solely enriches the expertise pool but additionally resonates with a broader buyer base. Companies that prioritize variety in their hiring practices report a surge in creativity and problem-solving capabilities, as groups composed of various backgrounds and experiences deliver diverse views to the desk.
Moreover, the transfer to recruit 22% extra candidates from non-elite universities challenges the normal norms of expertise acquisition, democratizing alternative and recognizing potential past prestigious diplomas.
This method not only involves taking part in discipline but also ignites a tradition of meritocracy and innovation inside the business. Public-private collaboration drives scalable change.
FAQs: Algorithmic Bias in 2025
Q1: What’s the distinction between bias and variance in AI?
A: Bias in AI refers to systematic errors that result in incorrect predictions or selections, typically because of flawed assumptions throughout the algorithm’s training phase.
Variance, then again, happens when an algorithm is just too delicate to the particular information it was skilled on, making it much less generalizable and probably overfitting to that information set.
In essence, whereas bias may be regarded as an algorithm’s lack of flexibility in studying from information, variance displays its overzealousness in adapting to the small print of the coaching information, at the expense of its efficiency on new, unseen information.
Both bias and variance are vital concerns in 2025, as they immediately influence the equity, reliability, and robustness of AI programs throughout numerous purposes. Bias is a systematic error favoring certain teams; variance is model sensitivity to coaching information.
Q2: Can open-source AI cut back bias?
A: Open-source AI has the potential to mitigate bias by fostering an extra varied and inclusive improvement environment. With a wider group of builders from totally different backgrounds contributing to the codebase, the algorithms may be scrutinized and improved upon from a number of views.
This collaborative method permits the identification and correction of biases that may not be evident to an extra homogenous group of builders.
Furthermore, the transparency inherent in open-source initiatives signifies that information units and decision-making processes may be brazenly audited, which is essential for constructing AI programs that can be truthful and equitable. Yes—group scrutiny exposes flaws (e.g., Hugging Face’s bias audits).
Q3: How does bias have an effect on healthcare AI?
A: Bias in healthcare AI can result in important disparities in patient outcomes. For instance, if an AI system is skilled predominantly on information from one demographic group, it might be much less correct when diagnosing situations in people from different demographics.
This not only undermines the effectiveness of medical interventions but may also erode belief in healthcare programs amongst underrepresented populations.
To mitigate such dangers, it’s important to include various information units and to repeatedly monitor AI purposes for biases that would have an effect on patient care. Models skilled on non-diverse information misdiagnose minorities (e.g., darker pores and skin rashes).
This autumn: Are all biases dangerous?
A: Not all biases are inherently dangerous; some are mandatory for the performance of AI programs. For instance, a spam filter is designed to be biased towards certain sorts of email content, which helps it serve its objective of defending customers from undesirable messages.
However, when biases result in unfair remedy or discrimination towards people or teams, they turn out to be problematic. It is essential to differentiate between practical biases that allow AI to carry out a selected job successfully and dangerous biases that perpetuate inequality or injustice.
Therefore, the main focus must be on figuring out and eliminating the latter, whereas sustaining the previous for the AI to function as supposed. No, however, unchecked ones harming marginalized teams require pressing fixes.
Q5: What can people do?
A: Individuals can play a pivotal role in shaping AI personalization to be extra equitable and simple. By actively offering suggestions to builders and corporations on situations of bias or unfair remedy, customers may help refine AI algorithms.
Additionally, people can educate themselves in regards to the underlying mechanisms of AI personalization, enabling them to advocate for transparency and accountability in AI programs.
Engaging in these practices not solely empowers customers but additionally places strain on the business to prioritize moral concerns in the event of AI technologies. Demand transparency, assist moral AI companies, and educate friends.

Conclusion: The Future is in Your Hands
As we navigate the ever-evolving panorama of AI personalization, it is clear that the ability to shape this future rests with every one of us. By staying knowledgeable and making acutely aware decisions, we will inform the trajectory of those applied sciences in the direction of a more inclusive and helpful horizon.
It’s as much as us to make sure that the AI of tomorrow displays the various wants and values of humanity, rather than the slender pursuits of a privileged few. Algorithmic bias isn’t inevitable—it’s a design flaw we will repair.
By 2025, the alternatives we make right this moment will decide whether or not AI empowers or oppresses. Audit your programs, advocate for justice, and bear in mind: ethics should code the long term.
Call to Action:
1: To actively form an equitable AI future, we should interact in a vigilant and ongoing technique of reviewing and refining the algorithms that underpin these programs. This calls for a dedication to transparency, permitting consultants and the general public alike to know how selections are made.
It additionally requires a diverse set of voices on the desk, making certain that the programs we construct replicate the rich tapestry of human expertise and don’t perpetuate historic injustices.
Only via collective effort and duty can we harness AI’s potential for the higher good, rather than the slender pursuits of a privileged few. Share this text to unfold consciousness.
2: In the realm of AI personalization, this collective effort translates into creating programs that can be inclusive, equitable, and clear. Developers and stakeholders should prioritize moral pointers that guarantee AI algorithms are free from biases that would hurt underrepresented teams.
By fostering an environment the place AI is developed with a consciousness of its societal influence, we will make sure that personalised experiences improve, rather than undermine, the material of our various societies. Join the Algorithmic Justice League’s campaigns.
3: To actually harness the ability of AI personalization, it’s crucial that we interact in moral information assortment practices, making certain that the data sets used are complete and inclusive of the various spectra of human experiences.
This means not solely gathering information from a variety of demographics but additionally respecting the privacy and consent of people whose information is being utilized.
By doing so, we will create AI programs that aren’t solely clever but additionally equitable and delicate to the nuances of human id and cultural variations, paving the way for expertise that adapts to serve everybody justly. Experiment with IBM’s AI Fairness 360 toolkit.
Discussion Question: To additional this endeavor, it is essential to interact in steady dialogue and collaboration amongst technologists, ethicists, and the broader group.
By incorporating various views and experiences, we will make sure that AI personalization doesn’t inadvertently perpetuate biase,s however as a substitute fosters inclusivity.
Tools just like the AI Fairness 360 toolkit are instrumental in this course of, providing a set of algorithms and metrics to assist builders detect and mitigate unfairness in their AI models. Should biased AI fashions be banned outright, or can they be reformed?